Tackling Gender Bias in AI: Practical Steps for Ethical AI Use | Insights & Best Practices
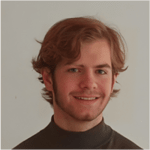
Salomon Kisters
Jun 26, 2023This post may contain affiliate links. If you use these links to buy something we may earn a commission. Thanks!
Artificial Intelligence (AI) is transforming our lives in ways we never imagined. From voice assistants to facial recognition, AI has emerged as a revolutionary technology that is reshaping industries and facilitating progress more than ever before. However, as AI becomes more widespread and indispensable, the issue of gender bias in AI has emerged as a growing concern.
According to recent studies, AI algorithms can inherit the biases of their human creators, leading to unequal treatment and discrimination against women and other marginalized groups. Indeed, it is not uncommon to find AI-powered systems that reinforce gender stereotypes, perpetuate sexism, and ultimately undermine the ethical principles that are essential in the development of AI.
To overcome this challenge, it is imperative that we tackle gender bias in AI, and promote responsible and ethical use of this technology. This blog post delves deep into the issue of gender bias in AI and highlights some of the practical steps that can be taken to mitigate the problem. So, whether you’re a developer, AI engineer, tech enthusiast, or anyone interested in this topic, keep reading for insights on how to address gender bias in AI.
Understanding Gender Bias in AI
Gender bias in AI refers to the unfair treatment or discrimination against individuals or groups based on their gender, which is perpetuated by AI-powered systems. This issue arises because AI algorithms can reflect and amplify the biases of their human creators. For instance, if a training dataset comprises predominantly male candidates, the AI system may develop an implicit bias towards men, leading to an unfair advantage or disadvantage. This bias can manifest in various forms, including gender stereotypes, underrepresentation, and marginalization.
Moreover, gender bias in AI can have serious consequences, particularly as this technology becomes increasingly ubiquitous. Gender bias can lead to unequal access to healthcare services, biased hiring practices, and a lack of diverse perspectives in decision-making. Additionally, AI-powered systems that perpetuate gender stereotypes can reinforce harmful societal norms and values, exacerbating gender inequality.
As such, it is crucial to understand the nature and impact of gender bias in AI to discover ways to address it effectively. Next, we will discuss the practical steps that can be taken to mitigate gender bias in AI and promote responsible, ethical use of this transformative technology.
Impact of Gender Bias
The impact of gender bias in AI is far-reaching and can have serious implications for individuals and society as a whole. One major consequence of gender bias in AI is the perpetuation of harmful gender stereotypes. When machines are trained on biased data sets, they can infer that certain traits, behaviors, or occupations are exclusively associated with a particular gender, leading to the perpetuation of traditional gender roles and expectations.
This can exacerbate gender inequality by reinforcing existing societal stereotypes and making it harder for people to break free from traditional gender roles. Furthermore, gender bias can lead to underrepresentation or marginalization of certain groups. For instance, women may be unfairly excluded from job opportunities, while men may be granted access to privileges and opportunities that are not available to women.
The impact of gender bias in AI can also be seen in the field of healthcare, where it can lead to unequal access to medical treatments and services. For example, a bias in the algorithm may skew the diagnosis towards a particular gender, leading to wrong treatment as the algorithm is not considering the patient as per their biological characteristics. This can have serious consequences for people’s health and wellbeing, and exacerbate existing health disparities.
Moreover, the impact of gender bias in AI can also affect decision-making in various industries and sectors. AI-powered systems that perpetuate gender stereotypes may make decisions that reflect biases rather than objective data. This can lead to unfair outcomes such as biased hiring practices, lack of diversity in decision-making, and discriminatory lending practices.
Root Causes of Gender Bias in AI
Addressing gender bias in AI requires a comprehensive approach that tackles the root causes of the problem. One key factor contributing to gender bias in AI is the lack of diversity in the tech industry. The industry is heavily male-dominated, which leads to a lack of diverse perspectives and experiences that are essential for creating unbiased, fair AI systems.
To address this issue, it is essential to increase the number of women and underrepresented groups in AI-related fields. This can be achieved by providing education and training opportunities, mentoring programs, and initiatives to encourage young girls to pursue careers in science, technology, engineering, and mathematics (STEM) fields.
Another factor that contributes to gender bias in AI is the use of biased or incomplete data sets. The data sets used to train AI systems are often biased and reflect existing societal stereotypes and prejudices. To ensure that AI systems are unbiased, it is crucial to ensure that the data used to train them is diverse, representative, and free from any biases or prejudices.
To achieve this, it is essential to involve diverse groups of people in the data collection process and use tools that can identify and mitigate biases in the data. Additionally, AI systems should be developed with transparency and accountability in mind, so that it is clear how they make decisions, and stakeholders can identify and address any issues that arise.
Finally, it is important to promote the ethical and responsible use of AI systems. This requires developing clear ethical guidelines and standards for AI development and use, and ensuring that these are adhered to by all stakeholders. It also requires ongoing monitoring and evaluation of AI systems to identify and address any biases or unintended consequences that may arise.
Best Practices for Reducing Gender Bias in AI
Reducing gender bias in AI is a complex process that requires a comprehensive and multifaceted approach. Here are some best practices that can help in reducing gender bias in AI:
1. Conduct Bias Testing:
Developers and practitioners should conduct regular bias testing to evaluate the performance of the AI models and identify any source of bias. Bias testing can help ensure that the AI system operates in a gender-neutral way.
2. Ensure Diversity in Data:
To reduce gender bias in AI, it is important to ensure that the data used in training is diverse and representative. Diverse data can ensure that the AI models produce unbiased results. As such, developers should use large and diverse datasets containing information from different regions and demographics.
3. Design Ethical Guidelines:
Developers must design ethical guidelines and standards for the development and use of AI systems. This will help promote fairness and ethical behavior, ensure accountability, and help prevent gender bias.
4. Implement Transparency:
Transparency is key in reducing gender bias in AI. Developers must clearly explain the functionality and outcome of the AI system, to help users understand how the system is making decisions. This will promote public trust in AI systems.
5. Diversify Development Teams:
Increasing diversity in development teams is essential in reducing gender bias in AI. Bringing more women and diverse groups into AI development can help bring varied perspectives, experiences, and insights to the table, leading to a more objective development process.
6. Monitor Output for Gender Bias:
Developers must continually monitor the output of AI systems to ensure there is no gender bias. Implementing monitoring processes can help identify any unintended biases that the system may produce and address them before the AI becomes a problem.
Conclusion
In conclusion, tackling gender bias in AI is just one aspect of the larger ethical concerns we need to consider as AI becomes more integrated into our lives. It is crucial that we continue to develop ethical guidelines and strategies to ensure that AI serves all of humanity in a fair and accountable manner. This requires collaboration between developers, policymakers, and the public, as well as ongoing research and development.
We need to make sure that AI systems are designed to be transparent and understandable so that people can question the decisions that are made by these systems. Additionally, we should strive towards using diverse and representative datasets to train AI systems, particularly in fields such as criminal justice, healthcare, and hiring, to prevent the potential for reinforcing and amplifying pre-existing biases.
We must remain vigilant and proactive in addressing ethical challenges as they arise. By doing so, we can ensure that AI technologies serve humanity in a positive and transformative way, promoting fairness and accountability while avoiding perpetuating existing power structures and inequalities. It is only through such efforts that we can build a future where AI benefits us a
Stay informed with the latest insights in Crypto, Blockchain, and Cyber-Security! Subscribe to our newsletter now to receive exclusive updates, expert analyses, and current developments directly to your inbox. Don't miss the opportunity to expand your knowledge and stay up-to-date.
Love what you're reading? Subscribe for top stories in Crypto, Blockchain, and Cyber-Security. Stay informed with exclusive updates.
Please note that the Content may have been generated with the Help of AI. The editorial content of OriginStamp AG does not constitute a recommendation for investment or purchase advice. In principle, an investment can also lead to a total loss. Therefore, please seek advice before making an investment decision.
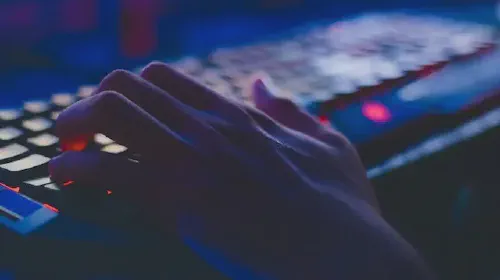
Buying Land in Decentraland: Step-by-Step Guide for Investors
Step-by-step guide for investors looking to buy land in Decentraland, the pioneering virtual world for gamers, producers, and entrepreneurs.
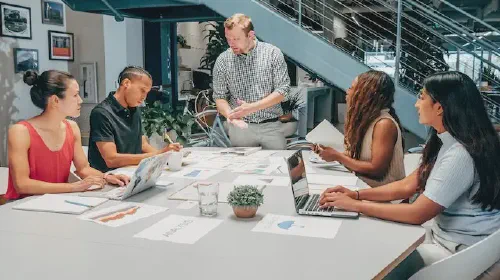
Enhancing Customer Loyalty with Digital Payments: Benefits & Strategies
Learn how digital payments can enhance customer loyalty by offering convenience, security, and personalized experiences. Explore strategies to implement seamless payment solutions.
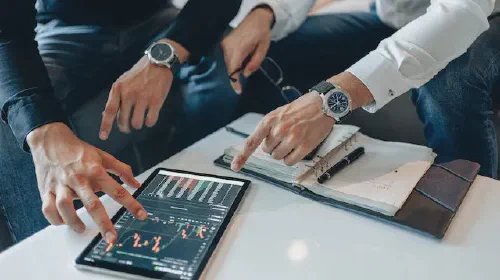
Fantom vs. Avalanche: Understanding the Variances
Discover the key differences between Fantom and Avalanche, two blockchain projects aiming to tackle scalability and interoperability challenges in the crypto space.
Protect your documents
Your gateway to unforgeable data. Imprint the authenticity of your information with our blockchain timestamp