What Is the Role of Edge Computing in Deep Learning?
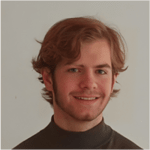
Salomon Kisters
Jul 11, 2023This post may contain affiliate links. If you use these links to buy something we may earn a commission. Thanks!
In today’s fast-paced digital world, deep learning has emerged as a powerful tool for solving complex problems and making intelligent decisions.
As the demand for artificial intelligence (AI) continues to grow, traditional centralized computing architectures face limitations due to latency, bandwidth constraints, and increasing volumes of data.
This is where edge computing comes into play, providing a distributed computing model that brings deep learning closer to data sources. In this blog post, we will explore the role of edge computing in deep learning and its practical applications.
Understanding Edge Computing
Edge computing is a decentralized computing paradigm that brings data processing to the edge of the network, near the data source or user device. Unlike traditional cloud computing, where data is sent to a centralized data center for processing, edge computing allows for real-time data analysis and decision-making at the edge of the network. This allows for reduced latency, improved performance, and enhanced data privacy.
Enhanced Real-time Decision Making
Deep learning algorithms require significant computational resources to train and make predictions. In scenarios where real-time decision making is crucial, such as autonomous vehicles or industrial automation, edge computing provides a viable solution.
By deploying deep learning models directly on edge devices or edge servers, we can reduce the latency associated with sending data to the cloud for processing. This enables faster decision-making and enhances the overall system’s responsiveness, thereby enabling time-critical applications.
Bandwidth Optimization
Deep learning models typically require large volumes of data for training and inference. Transmitting this data to centralized cloud servers can be challenging, especially when dealing with limited bandwidth or intermittent network connectivity.
In such cases, edge computing proves beneficial by minimizing the need to transfer massive amounts of data to the cloud. Instead, data is processed locally on edge devices or at edge servers, reducing the bandwidth requirements and reliance on a stable network connection.
This optimization not only mitigates the risk of data loss but also lowers the operational costs associated with transmitting large datasets.
Data Privacy and Security
The sensitive nature of data in deep learning applications necessitates robust privacy and security measures. Edge computing addresses these concerns by keeping sensitive data within the boundaries of the local network, reducing the exposure to potential security breaches or data leaks.
By performing data processing and analysis on the edge, personal and confidential information can be kept confidential, ensuring compliance with data privacy regulations.
Real-time Analytics at Scale
Edge computing allows for distributing intelligent data analytics at scale across a network of edge devices. Each device can independently analyze local data, make predictions, and leverage collective intelligence to generate insights. This distributed approach to deep learning enables near real-time analysis of data from multiple sources simultaneously.
For example, in a smart city scenario, edge devices located across the urban landscape can monitor and respond to various environmental factors, ensuring efficient resource allocation, improving traffic flow, and enhancing overall city operations.
Practical Applications of Edge Computing in Deep Learning
Now that we understand the key benefits of edge computing in deep learning, let’s explore some practical applications where this technology finds great value:
Internet of Things (IoT)
The proliferation of IoT devices generates an enormous amount of data that needs to be processed and analyzed. Edge computing enables efficient data analysis closer to the source, reducing network congestion and improving response times for critical IoT applications.
For instance, in a smart home, edge devices such as home security systems or voice assistants can process audio and video data locally to identify potential security threats or provide instant voice-based responses.
Autonomous Vehicles
The success of autonomous vehicles heavily relies on real-time data processing and decision-making. Edge computing facilitates the deployment of deep learning algorithms directly onto the vehicles or edge servers.
This enables cars to analyze sensor data, recognize objects, and make driving decisions without relying solely on cloud-based processing. By minimizing latency in perception, decision-making, and control, edge computing significantly contributes to the safety and performance of self-driving vehicles.
Healthcare
In the healthcare sector, edge computing presents numerous benefits in terms of patient monitoring, diagnostics, and personalized treatment. Wearable devices, such as smartwatches or health trackers, can process vital signs locally and respond in real-time.
This immediate analysis and processing allow for proactive interventions and timely alerts, ensuring the well-being of patients. Additionally, edge computing enables the aggregation and analysis of large medical datasets, creating opportunities for deep learning models to accelerate medical research and diagnosis.
Industrial Automation
Edge computing plays a critical role in industrial automation by enabling real-time control, monitoring, and decision-making. In manufacturing facilities, edge devices can process sensor data to detect anomalies, predict maintenance needs, and optimize production processes.
By deploying deep learning models at the edge, manufacturers can increase productivity, reduce downtime, and improve overall operational efficiency while ensuring data privacy and security within the company’s infrastructure.
Video Surveillance and Security
Edge computing, coupled with deep learning, transforms conventional video surveillance systems into proactive and intelligent security solutions. By analyzing video footage at the edge, surveillance cameras can identify suspicious activities, detect unauthorized access, and raise alerts in real-time.
This localized processing minimizes response times, improves accuracy, and reduces the bandwidth requirements for transmitting video data to a centralized control center. Moreover, it enhances privacy by lowering the need for constant live video feeds.
Conclusion
Edge computing plays a pivotal role in extending the capabilities of deep learning in various domains. By moving computation closer to data sources, edge computing minimizes latency, optimizes bandwidth usage, enhances data privacy and security, and facilitates real-time decision-making. Its practical applications encompass diverse fields such as IoT, autonomous vehicles, healthcare, industrial automation, and video surveillance.
As the demand for deep learning continues to surge, edge computing will undoubtedly remain a critical enabler in unlocking the full potential of intelligent systems. Embracing edge computing in conjunction with deep learning empowers organizations to leverage real-time insights, improve efficiency, and shape the future of AI-driven technologies.
Stay informed with the latest insights in Crypto, Blockchain, and Cyber-Security! Subscribe to our newsletter now to receive exclusive updates, expert analyses, and current developments directly to your inbox. Don't miss the opportunity to expand your knowledge and stay up-to-date.
Love what you're reading? Subscribe for top stories in Crypto, Blockchain, and Cyber-Security. Stay informed with exclusive updates.
Please note that the Content may have been generated with the Help of AI. The editorial content of OriginStamp AG does not constitute a recommendation for investment or purchase advice. In principle, an investment can also lead to a total loss. Therefore, please seek advice before making an investment decision.
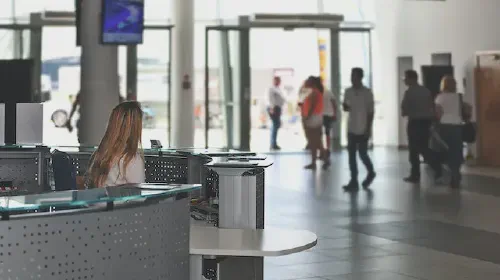
The Journey of Digital Payment Transactions: Evolution, Benefits, Challenges, and Future Trends
Explore the evolution, benefits, and challenges of digital payment transactions, from cash to cryptocurrency, and discover the future trends in the digital payment industry.
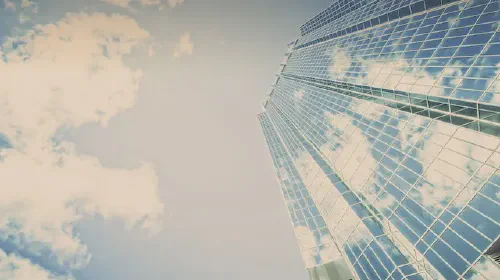
Edge Computing vs Distributed Computing: Key Differences Explained
Understand the key differences between Edge Computing and Distributed Computing, their architectures, use cases, and how they enhance modern applications.
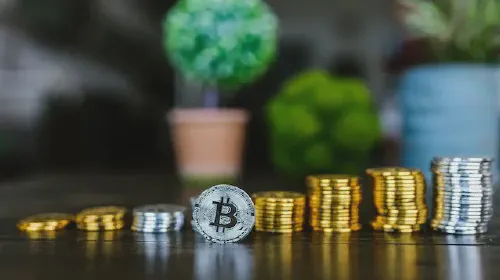
What Students Should Know About Cryptocurrency: Key Information and Risks
Key information and risks that students should know about cryptocurrency to make informed decisions and avoid potential pitfalls in the volatile market.
Protect your documents
Your gateway to unforgeable data. Imprint the authenticity of your information with our blockchain timestamp