What'S The Connection Between Quantum Computing And Machine Learning?
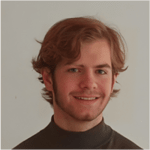
Salomon Kisters
Jul 13, 2023This post may contain affiliate links. If you use these links to buy something we may earn a commission. Thanks!
Quantum computing and machine learning are two rapidly advancing fields with the potential to revolutionize industries and open up new possibilities for scientific breakthroughs.
At first glance, it may seem that these two areas of research are unrelated. However, closer examination reveals a deep connection between quantum computing and machine learning that has the potential to transform the way we approach complex problems.
In this blog post, we will explore the fascinating intersection of quantum computing and machine learning, and reveal the exciting possibilities that arise from their integration.
Quantum Advantage in Machine Learning
Quantum computing offers unique advantages that have the potential to significantly enhance machine learning algorithms.
One of the primary benefits is exponential parallelism, where quantum computers can process large amounts of information simultaneously, unlike classical computers that perform calculations sequentially. This immense processing power opens up the door to solving complex problems that were previously intractable due to their size or computational complexity.
Quantum machine learning algorithms can leverage this parallelism to speed up tasks such as pattern recognition, optimization, and data classification.
For example, quantum algorithms like quantum support vector machines (QSVM) have demonstrated the ability to classify data sets exponentially faster than classical counterparts. By harnessing the power of quantum computing, machine learning algorithms can achieve unprecedented speed and efficiency, offering us the potential for breakthroughs in various applications.
Enhancing Data Analysis through Quantum Computing
Data analysis is critical in machine learning, providing insights from vast amounts of information to make informed decisions. Quantum computing has the potential to revolutionize data analysis by enabling us to extract patterns and gain insights from increasingly complex and large datasets. Traditional machine learning algorithms, such as clustering and classification, can be significantly enhanced by leveraging quantum techniques.
Quantum algorithms, such as amplitude estimation and quantum principal component analysis (PCA), offer more efficient ways to perform data analysis tasks. The ability of quantum computers to store and process vast amounts of information simultaneously allows us to explore higher-dimensional data spaces and uncover hidden patterns that may have gone unnoticed using classical methods.
As a result, quantum machine learning algorithms offer the potential to extract more accurate and meaningful insights from data, providing a significant advantage in decision-making processes.
Quantum Machine Learning Models
Another exciting development at the intersection of quantum computing and machine learning is the emergence of quantum machine learning models. These models utilize the principles of quantum mechanics to enhance traditional machine learning algorithms and address specific challenges in the field.
Quantum variants of popular machine learning models, such as quantum neural networks and quantum variational classifiers, aim to improve accuracy and performance.
Quantum neural networks, for instance, exploit the unique properties of quantum systems to process and represent information more effectively. By combining classical and quantum elements, these networks offer the potential for increased learning capabilities and improved performance in certain applications.
Similarly, quantum variational classifiers leverage quantum algorithms to enhance their ability to solve complex classification problems. The development of these quantum machine learning models opens up new avenues for research and innovation in the field of machine learning.
Quantum Machine Learning Hardware
Hardware plays a crucial role in the implementation of quantum computing and machine learning systems. Advancements in quantum hardware have paved the way for the practical realization of quantum machine learning algorithms. Quantum processors, such as superconducting qubits, are becoming more accessible, reliable, and scalable, allowing researchers and organizations to experiment with quantum algorithms for machine learning applications.
Investments in quantum hardware research and development promise to drive further progress and innovation in the field. As the field continues to evolve, we can expect more powerful hardware that will enable faster and more accurate computations in quantum machine learning. The development and optimization of quantum hardware are essential to unlock the full potential of quantum computing and facilitate its integration with machine learning techniques.
Challenges and Future Outlook
While the integration of quantum computing and machine learning holds immense promise, there are several challenges that need to be addressed.
Quantum computers are still in their early stages of development, and practical quantum machine learning algorithms are limited in their applicability. The noise and fragility of quantum systems pose significant challenges in implementing and scaling quantum algorithms for machine learning tasks.
However, the rapidly evolving field of quantum error correction and fault-tolerant quantum computation holds the potential to overcome these challenges. Continued research and innovation in these areas will pave the way for the practical utilization of quantum computing in machine learning applications.
As quantum hardware improves and quantum algorithms become more robust, we can anticipate a future where quantum machine learning becomes an integral part of various industries, delivering insights and discoveries that were previously unimaginable.
Conclusion
In conclusion, the connection between quantum computing and machine learning is multifaceted and holds tremendous potential for advancing both fields.
Quantum computing’s exponential parallelism and enhanced data analysis capabilities offer significant advantages for machine learning algorithms. Quantum machine learning models and hardware advancements further solidify the relationship between these two domains, paving the way for exciting future innovations.
As the integration of quantum computing and machine learning continues to evolve, it is crucial to address the challenges and overcome the limitations that currently hinder widespread adoption.
With ongoing research and development efforts, we can expect quantum machine learning to transform industries, accelerate scientific advancements, and tackle complex problems that were previously unfathomable.
The future of quantum computing and machine learning is undoubtedly interconnected and full of boundless opportunities.
Stay informed with the latest insights in Crypto, Blockchain, and Cyber-Security! Subscribe to our newsletter now to receive exclusive updates, expert analyses, and current developments directly to your inbox. Don't miss the opportunity to expand your knowledge and stay up-to-date.
Love what you're reading? Subscribe for top stories in Crypto, Blockchain, and Cyber-Security. Stay informed with exclusive updates.
Please note that the Content may have been generated with the Help of AI. The editorial content of OriginStamp AG does not constitute a recommendation for investment or purchase advice. In principle, an investment can also lead to a total loss. Therefore, please seek advice before making an investment decision.
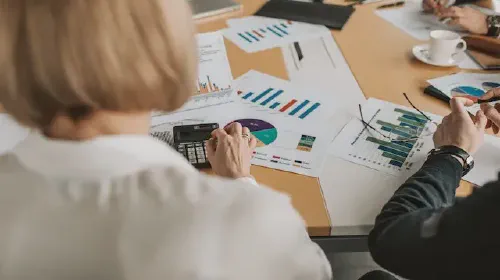
Contactless Cards vs Mobile Wallets: A Battle?
Discover the differences and similarities between using contactless cards versus mobile wallets in today's payment landscape. Explore the benefits, security features, and future trends in this comprehensive comparison.
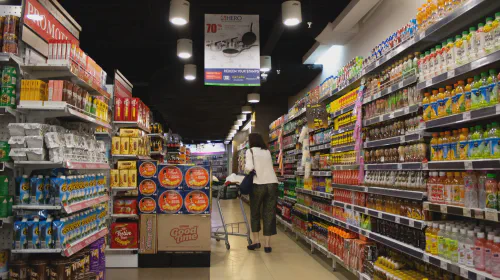
5 Benefits of Blockchain in Food Supply Chains
Learn about the 5 key benefits of using blockchain technology in food supply chains, including enhanced transparency, traceability, and reduced food fraud.
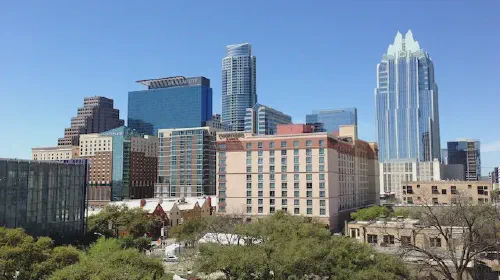
Understanding Ethical Hacking: Is It Truly Ethical?
Explore the concept of ethical hacking and whether it truly deserves its label of being ethical. Learn about the practice, arguments for and against, risks and benefits, legal landscape, and more.
Protect your documents
Your gateway to unforgeable data. Imprint the authenticity of your information with our blockchain timestamp